Licensed for Distribution
Published 13 December 2021 - ID G00740723
By Henry Cook, Merv Adrian, Rick Greenwald, Adam Ronthal, Philip Russom
Database management systems continue their move to the cloud — a move that is producing an increasingly complex landscape of vendors and offerings. This Magic Quadrant will help data and analytics leaders make the right choices in a complex and fast-evolving market.
Strategic Planning Assumptions
By 2025, cloud preference for data management will substantially reduce the vendor landscape while the growth in multi-cloud will increase the complexity for data governance and integration.
By 2022, cloud database management system (DBMS) revenue will account for 50% of the total DBMS market revenue.
Market Definition/Description
Gartner defines the cloud DBMS market as follows. Core capabilities are that vendors fully supply provider-managed public or private cloud software systems that manage data on cloud storage. Data is stored in a cloud storage tier (such as a cloud object store, distributed data store or other proprietary cloud storage infrastructure). Optionally, they may cater to multiple data models and data types — relational, nonrelational (document, key value, wide column, graph), geospatial, time series and others.
These DBMSs reflect optimization strategies designed to support transactions and/or analytical processing for one or more of the following use cases:
Traditional and augmented transaction processing
Traditional and logical data warehouse
Data science exploration/deep learning
Stream/event processing
Operational intelligence
This market does not include vendors that only provide DBMSs hosted in infrastructure as a service (IaaS), such as in a virtual machine or container, and managed by the customer.
Magic Quadrant
Figure 1. Magic Quadrant for Cloud Database Management Systems
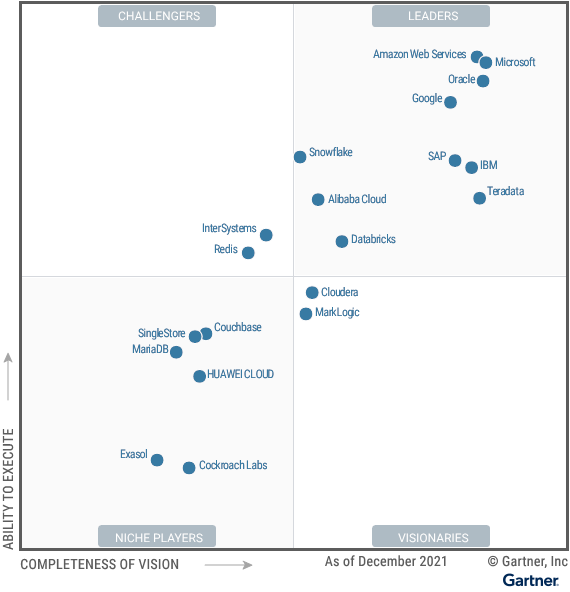
Source: Gartner (December 2021)
Vendor Strengths and Cautions
Alibaba Cloud
Alibaba Cloud is a Leader in this Magic Quadrant. Its products are broadly focused on operational and analytical databases plus a wide range of other cloud-based services. Its operations are primarily based in China and Asia, but it also has an expanding presence in Europe and North America. Its international headquarters is in Singapore.
Operational database services include PolarDB (compatible with MySQL, PostgreSQL and Oracle), PolarDB-X, RDS and MyBase. Analytics database services include AnalyticDB, MaxCompute, Data Lake Formation and E-MapReduce (EMR).
Strengths
Wide Range of Offerings: The vendor offers multiple products, even within a category. For example, there are multiple operational database products in addition to PolarDB and two flavors of AnalyticDB. One is based on Greenplum, and the other is natively developed for large-scale, high-concurrency OLAP-style processing.
Innovation and Scale: PolarDB, AnalyticDB and AliSQL were designed and developed in-house and are web-scale cloud products. PolarDB powers the Alibaba Double 11 Global Shopping Festival, which in 2020 handled peak processing of 583,000 orders per second and set a new record with 140 million queries per second during peak time.
Expansion Into Asia, Europe and Latin America: With private funding and international and Alibaba Cloud headquarters in Singapore, Alibaba Cloud continues building a presence in the global market reaching out from its home in China, where it is the largest cloud provider. Many global companies needing to use a cloud provider in China find Alibaba to be a good fit.
Cautions
Limited Presence in Europe and North America: Alibaba Cloud has made good progress in expanding beyond China. However, it still does not have the same level of presence in Europe and the Americas as other large cloud providers.
Overlapping Offerings: Although a strength in one respect, Alibaba Cloud’s wide range of products can still be a source of confusion. However, its documentation in English and other languages is easy to access and navigate, and Alibaba Cloud has made significant progress in streamlining unnecessary overlaps in its products.
Geopolitics: Although clearly not something it can control, the potential for trade wars and sanctions could hinder Alibaba Cloud’s plans. It is worth noting, however, that Alibaba is self-reliant in terms of technology supply, and AliCloud is headquartered outside China.
Amazon Web Services
Amazon Web Services (AWS) is a Leader in this Magic Quadrant. It offers a range of database management services. Some are aimed at operational use cases, as is the case with Amazon Relational Database Service (RDS), Amazon Aurora and Amazon DynamoDB, for example. Others are aimed at analytics use cases, as is the case with Amazon Redshift, Amazon Athena and Amazon EMR. AWS also offers memory-intensive services and offerings aimed at specialized use cases. AWS is able to offer infrastructure-level capabilities to cloud DBMS with, for example, its AWS Nitro System, AQUA (Advanced Query Accelerator) for Amazon Redshift and hardware-assisted Redshift RA3 nodes.
AWS is the largest cloud service provider in the world by revenue, with an international presence and a global client base across all major industries.
Strengths
Leading Market Presence: AWS is the world’s largest cloud database service provider by revenue. It has offered production cloud services for more than 10 years, with tens of thousands of customers, which also makes it the most mature cloud provider in terms of offerings and capabilities.
Breadth of Services: AWS offers more than a dozen different database services, and reviewers on Gartner Peer Insights express appreciation with the breadth of functionality that the combined offerings deliver. In addition, AWS has a very broad base of data centers, providing lower latency based on proximity across the world.
Performance: Reviewers on Gartner Peer Insights praise multiple AWS services for their performance: Amazon Aurora, Amazon Redshift and Amazon DynamoDB, which handles billions of transactions per day for all of AWS’s client services. The length of time that AWS database services have been in production has led to growth in workloads and improved the ability of AWS to handle these more mature workloads. In addition, AWS’s uptime and reliability is the most reliable of the vendors evaluated in this research.
Cautions
AWS Centric-Focus: AWS is the largest cloud vendor. However, it is behind some CSPs and virtually all ISVs in offering its services on multiple cloud platforms. This centricity appears to be easing slightly, given AWS’s support for hybrid cloud environments, the sharing of its data among different clouds in certain scenarios, and a data strategy that acknowledges and is adapting to the existence of multiple cloud platforms.
Integration Across Portfolio: AWS uses a best-fit approach to database services, which enables it to deliver focused solutions for different types of use cases. However, AWS data integration, catalog and metadata solutions are emerging and, today, are not as strong as some competitors’, which can lead to more work for architects.
Cost of Management: AWS delivers a deep and rich toolkit for users to assemble optimal solutions. This some-assembly-required approach can sometimes lead to somewhat more management overhead and cost being required.
Cloudera
Cloudera is a Visionary in this Magic Quadrant. Cloudera Data Platform (CDP), which includes CDP Data Hub, CDP DataFlow, CDP Data Engineering, CDP Operational Database, CDP Data Warehouse and CDP Machine Learning, offers operational and analytics uses. Cloudera Workload XM is used to migrate, analyze, optimize and scale workloads. Cloudera Shared Data Experience (SDX) provides intercloud and multi-cloud unified security, governance and metadata management.
Cloudera’s global operations are strongest in North America and Europe, mainly in financial services, healthcare, retail and utilities. Recent investments focus on delivering cloud-native services, enhancing operational DBMS, and some residual work on integrating and aligning the former Cloudera and Hortonworks offerings.
Strengths
On-Premises Base: Among its 2,000 customers, Cloudera has significant on-premises deployments. It continues to support and update these during the transition to its CDP platform and the ongoing movement of customers to the cloud. Its hybrid architecture using SDX for management and governance will smooth this transition.
Open-Source Basis: Cloudera is a leading committer in many of its 30+ open-source components such as Apache Hive, Impala, HBase, Phoenix, Spark, Flink and Kafka. Cloudera markets them within its offerings under the Apache license, using the open-source AGPL license for its own components.
Hybrid, Multi-cloud and Intercloud Deployment: Cloudera’s focus on hybrid cloud deployments is based on creating a governed architecture designed for deployments on-premises and on multiple public clouds. It gives customers a way to move appropriate workloads to the cloud in a measured way at their own pace, while retaining and extending policy enforcement and security.
Cautions
Competition for Multiple Use Cases: Cloudera faces different competitors in different use cases. Some use many of the same open-source components in their offerings. However, Cloudera’s ability to support multiple projects from the same integrated platform and the same data context provided by SDX is useful.
Phased Rollout of CDP: The magnitude and complexity of the change from the old products to the new ones continues to dominate client inquiries received by Gartner. Basic cluster management for all analytics is available on AWS and Azure, but some CDP offerings were still on Cloudera’s roadmap for delivery during the next three to six months in mid-2021.
New Pricing Can Be Costly: The compute and storage cloud-based pricing model is new to Cloudera’s on-premises customers. Gartner’s conversations with clients have often centered on complaints about higher list prices for the legacy Hortonworks Data Platform (HDP). In these discussions, clients also often complain about additional variable pricing for computing and storage resources for new, larger nodes, especially when heavily discounted multiyear deals are expiring. Cloudera has discounted new deals to existing customers to help make the pricing transition more gradual.
Cockroach Labs
Cockroach Labs is a Niche Player in this Magic Quadrant. It is active in multiple markets including finance and retail. It offers CockroachDB Dedicated, available on AWS and Google Cloud Platform (GCP). CockroachDB Dedicated is a managed single-tenant offering. CockroachDB Serverless is a multitenant serverless offering.
CockroachDB Dedicated is specifically architected for use cases that require write operations across geographically distributed systems with full consistency.
Strengths
Distributed Transactions: CockroachDB Dedicated is designed to provide the ability to receive updates across distributed platforms with very low latency. Some use cases, such as global finance transactions, require this type of transaction with the full consistency offered by CockroachDB Dedicated.
Multicloud, Intercloud and Hybrid: CockroachDB Dedicated runs on both AWS and GCP, and hybrid on-premises, as well as accessing data on multiple clouds, which some distributed competitors cannot offer.
Node-Based Pricing: CockroachDB Dedicated offers predictable, node-based pricing.
Cautions
Niche Requirement: Although some use cases absolutely require a distributed transaction capability with the full consistency offered by CockroachDB Dedicated, these use cases are in the minority. Other, more mature transactional services have a richer feature set outside of this niche.
Expertise Required: CockroachDB Dedicated offers the ability to implement distributed transactions with ACID properties, and this is a challenging task for any database technology. Implementing the system properly and understanding how this is done requires particular expertise.
Competition From CSPs and Other Niche Vendors: Recently, other vendors both in the U.S. and China are offering similar capabilities, making a niche market more competitive. This provides more choice for the using organization, but also complicates the evaluation in this new part of the market. However, none of those other solutions are included in this research.
Couchbase
Couchbase is a Niche Player in this Magic Quadrant. It is a new entrant. Its multi-model DBMS has a heritage in high-performance nonrelational operational databases, with a more recent focus on relational capabilities such as SQL, schema and transactions, deepening hybrid analytical use cases, and extending cloud provider support. Its operations are primarily based in North America, but it has a substantial presence in Europe and growth in the Asia/Pacific region. It is broadly represented across major market sectors.
Much of Couchbase’s roadmap is targeted toward enhancing its real-time analytics, extending cloud capabilities and delivering availability on leading cloud platforms. It does not currently position itself for use in data warehouses or data lakes, but many customers are using its analytics service for operational intelligence.
Strengths
High-Performance Multimodel Capabilities: Couchbase has significant numbers of on-premises and self-managed cloud deployments in use for demanding in-memory workloads in large enterprises. Its ability to combine a document-based architecture with in-memory processing and ACID transactions, using a popular SQL-based query language, makes it suitable to address a growing demand for such applications.
Broad Deployment Choices: Couchbase made mobile and edge-based deployment a priority early, and offers a mature replication capability that enables systems that span on-premises, edge, peer-to-peer inter-device synchronization, and both public and private cloud. Support of mobile is very uncommon among DBMSs.
Positive Customer Sentiment: Couchbase is described favorably in inquiries to Gartner, and is often seen as an alternative to MongoDB — half of the Gartner Peer Insights reviews for Couchbase considered MongoDB as an alternative. Couchbase is evaluated favorably on pricing and contract flexibility, a frequent topic in competitive discussions.
Cautions
Enterprise Requirements: Couchbase lagged in addressing high-performance requirements such as workload management and automated performance tuning. These will need to be enhanced for larger-enterprise-footprint deployments. Recent additions to the database include cost-based query optimization, collection-level query and index processing. Couchbase also offers Autonomous Operator for Kubernetes, which supports autoscaling for self-managed deployments.
Missing Some Analytics Features: Couchbase has added significant analytics capabilities, but still needs to enhance its distributed access feature set to be used more broadly in modern data warehouse and lake-house-style deployments.
Relatively Low Market Awareness: Couchbase has an enthusiastic audience among developers, but has not been freely available in the cloud until recently, and remains relatively little-known among executives. This may make it difficult to gain approval for its use without aggressive sponsorship and effective proof-of-concept management.
Databricks
Databricks is a Leader in this Magic Quadrant. It offers Databricks Lakehouse Platform on Microsoft Azure (Azure Databricks), AWS, Alibaba and GCP. Lakehouse Platform consists of data stored in a data lake, including open-source formats, that can also be used through Delta Lake, which adds metadata and structures to the underlying data to deliver some of the capabilities of a traditional data warehouse.
Databricks also offers Solution Accelerators, which are preconfigured notebooks aimed at specific use cases and industries.
Strengths
Lakehouse Concept and Implementation: Databricks is a prime advocate for the lake-house concept, which combines the capabilities of a data lake and a data warehouse operating on the same data. This architecture has been taken up by the market, and Delta Lake implements the architecture in a way that leads the marketplace at this time.
Openness: Databricks offers a truly open architecture, even supporting open-source formats for storage. This openness extends to the broad reach in connectivity with other data sources on a wide variety of platforms and helps to broaden the reach and attractiveness of the vendor’s data marketplace. Databricks is also driving the open-source Delta Sharing Initiative in collaboration with other vendors.
Scalability: Gartner Peer Insights reviewers have praised the solution’s scalability, and Gartner has also talked with users who successfully run extremely challenging workloads. Some customers have petabytes of storage in their systems.
Cautions
Relational Capabilities: The lake-house architecture provides relational capabilities on top of data lake storage. The advanced relational capabilities, although good, are not up to the level of more-mature relational databases.
Workload Management: Databricks has added to its workload management capabilities with the latest release, but its capabilities are still not equal to the more-mature solutions on the market.
Increasing Competition for the Lakehouse Space: The lake-house concept is gaining traction with customers as well as most of the major CSPs in this space, so Databricks will have to contend with significant competitors over the next few years.
Exasol
Exasol is a Niche Player in this Magic Quadrant. It is headquartered in Nuremberg, Germany, and is a new entrant this year. It provides the Exasol database, an in-memory analytics database. Exasol is a specialist vendor focused solely on analytics database solutions such as data warehouse and data lake. Historically, most of its business has been in Europe, but it now has a presence in North America and the Middle East.
Exasol has long experience in data warehousing, starting as an on-premises product. Its product provides the main features that customers in this market expect, such as in-memory, columnar, data lake integration and the ability to work with machine learning (ML).
Strengths
Focus: Historically, Exasol has focused mainly on its analytics database system. It does not position itself as a transactional database. The Exasol DBMS provides the main modern functions expected of a product for analytical use cases, such as integration with data lakes, ML, in-memory column stores, etc. It caters to organizations that require these targeted requirements and do not wish to incur the complexity of further services. The vendor is also committed to expanding its product portfolio, through the acquisition of yotilla, to provide automated business intelligence (BI) tool capabilities.
Experience: Exasol has been in existence as a specialist analytical data warehouse DBMS for over 20 years. It applies this experience to its technology, and to helping its customers gain benefit. While its database started on-premises, it has been available in the public cloud since 2015, where most new customers start today. Exasol has brought this experience with it to its new cloud customers.
Price and Performance: A central message of the vendor’s marketing focuses on price and performance. Exasol is a good option for consideration by organizations seeking a straightforward and cost-effective solution.
Cautions
Company Size: Exasol is one of the smaller vendors in the Magic Quadrant. However, it has a long track record, is profitable and has successfully raised capital in an IPO for further expansion. It has been evaluated and is used by Fortune 100 corporations.
Single Product: Previously, Exasol focused on providing a single product, its in-memory analytics DBMS. This was less attractive to organizations that wished to purchase a wider variety of ancillary products. However, for organizations that simply wished to acquire an analytical database, without the additional complexity of being sold a wide range of other products, this was appealing. More recently, Exasol has sought to widen its range of products, in particular by the acquisition of yotilla for BI and data warehouse automation.
Partner Functionality: Some of the more-specialized features are provided through partners rather than being native to the database, and therefore may be charged separately.
Google is a Leader in this Magic Quadrant. Its Google Cloud Platform supports many database platform as a service (dbPaaS) products, from fully managed versions of products from third-party providers to its own products, namely Google Cloud SQL, Cloud Spanner, Cloud Bigtable, BigQuery, Dataproc, Cloud Firestore and Firebase Realtime Database. They address both transactional and analytics use cases.
Google’s focus on openness is shown by products such as BigQuery Omni, a multi-cloud offering that enables GCP customers to access data via BigQuery on other CSPs’ platforms. It also recently announced Google Dataplex, which serves as the basis for GCP’s data fabric and unified data ecosystem vision and became generally available in October 2021.
Strengths
Augmented Data Management Focus and Ecosystem Vision: The recently announced Google Dataplex provides unification across GCP data services with automatic data discovery, metadata harvesting, life cycle management, data quality, integration and governance capabilities. GCP is leveraging artificial intelligence (AI) and ML capabilities to augment this ecosystem as much as possible. Google is a leader in the AI and ML space (for example, with TensorFlow).
Open Cloud Infrastructure With Modern Capabilities: GCP provides a modern cloud environment. Most offerings are serverless, and Google’s Colossus data store provides a common framework for data, enabling features such as federated queries between Spanner and BigQuery. Further, GCP is pursuing a strategy of openness and has started allowing easy access and consumption of data in other clouds through products like BigQuery Omni.
Differentiated Datasets and Growing Vertical Industry Focus: GCP provides easy integration and consumption of proprietary Google datasets such as Google Trends, Google Maps, Google Search, Google Ads and others. GCP is also investing in streamlined, vertical industry solutions with a dedicated and growing industry-oriented sales force.
Cautions
Less Diverse Portfolio Than CSP Competitors: While addressing the majority of core requirements, GCP’s portfolio is less diverse and comprehensive than competitive cloud service providers. Strong technology partnerships do fill some of these gaps, but prospective customers should be aware of what are third-party integrations rather than native GCP offerings.
Hybrid Experience Is Lacking: GCP does not have a production hybrid environment with Anthos, but end users report that the local development experience is not fully reflective of core capabilities in the cloud.
Evolving User Experience: Reviewers of GCP on Gartner Peer Insights report issues with ease of use with the UI, adopting new features, capacity planning and cost management. These are all areas that GCP is actively working on.
HUAWEI CLOUD
HUAWEI CLOUD is a Niche Player in this Magic Quadrant. Offerings available on Huawei CLOUD and Huawei CLOUD Stack (for on-premises deployment) include relational GaussDB(for openGauss), GaussDB(for MySQL), GaussDB Data Warehouse Service, nonrelational GaussDB(for Mongo), GaussDB(for Influx), GaussDB(for Cassandra), GaussDB(for Redis) and Hadoop-based big data platform FusionInsight.
HUAWEI CLOUD’s operations are primarily in the Asia/Pacific region, with some presence in the Middle East, Africa and Latin America. Public administration, telecom, and finance and insurance account for over half of the vendor’s cloud DBMS industry penetration. The vendor is focused on providing a comprehensive hybrid stack for the full range of data management use cases.
Strengths
Industry Strength: Huawei is a multinational corporation, primarily known for its large 5G telecommunications infrastructure. The data management capabilities, just one of many product lines it offers, were built to manage Huawei’s substantial global industry businesses in that area.
Strength in Asia: Huawei’s business is largely focused on China. The vendor has proven itself at scale in one of the more demanding global markets. China-based deployments tend to operate on a large scale and require significant core capabilities.
Breadth of Cloud Portfolio and Ecosystem: Huawei has built a comprehensive and robust portfolio that can support almost all the use cases we have defined for this market. Many of its products are tightly integrated with its cloud infrastructure to provide enhanced performance and reliability.
Cautions
Confusing Portfolio: Although Huawei has a broad range of offerings, it may be difficult for prospective customers to determine which solution is the best choice, as there are many overlaps — for example, GaussDB(for MySQL) overlaps with RDS for MySQL and RDS for PostgreSQL. We expect Huawei’s go-to-market approach to improve as GaussDB plays a more prominent role.
Geopolitical Concerns: The geopolitical situation will continue to make it challenging for Huawei to market its products effectively in North America and Europe. The vast majority of Huawei’s business remains in the Asia/Pacific region.
Inconsistent Delivery and Marketing: Customers visiting the international version of Huawei’s website will see different offerings from those visiting the Chinese version. The English language pages of HUAWEI CLOUD do not yet cover GaussDB or some of the value-added components that Huawei has built.
IBM
IBM is a Leader in this Magic Quadrant. Its offerings coalesce around Cloud Pak for Data, a unified integration layer for containerized DBMS services built on Red Hat OpenShift. Cloud Pak for Data serves as a platform for many other IBM data management offerings, including IBM Db2 on Cloud, IBM Db2 Warehouse on Cloud, IBM Cloud SQL Query, IBM Cloudant, the IBM Cloud Database family and IBM Event Streams, plus managed services for third-party offerings. The IBM Cloud Database family provides a variety of managed data technologies, including PostgreSQL, MongoDB, Elasticsearch, Redis, RabbitMQ, DataStax, EnterpriseDB and etc. IBM Db2 can also run on IBM Cloud, AWS and Microsoft without Cloud Pak for Data.
IBM operates globally, and in all industries and organization sizes. IBM invests broadly in leading data management technologies. It addresses both operational and analytical use cases in this Magic Quadrant.
Strengths
Multi-cloud/Hybrid Ecosystem Vision: IBM’s Cloud Pak for Data represents a cohesive ecosystem with a broad range of data management capabilities including multiple DBMS offerings, data integration, analytics, data science, metadata and governance. Built on OpenShift Kubernetes, it is a transportable environment that can be deployed in the customer’s choice of public cloud, or on-premises in software or an appliance form factor. In addition to IBM’s DBMS offerings (Db2, Netezza, Cloudant), Cloud Pak for Data also supports third-party offerings like DataStax, MongoDB, Redis and others. IBM provides mature data virtualization capabilities through IBM Data Federation, which is packaged as part of Cloud Pak for Data. Db2 also runs on IBM Cloud, AWS and Azure, independent of Cloud Pak for Data.
Metadata and Governance Capabilities: IBM has always been strong in metadata management and governance capabilities. IBM Watson Knowledge Catalog is included in Cloud Pak for Data and can provide governance for all data assets under management spanning multiple clouds. This complements their data integration capabilities, including batch, streaming and virtualization.
AI and ML Augmentation: IBM is focused on infusing AI and ML capabilities throughout the Cloud Pak for Data platform. Db2 on Cloud and Db2 Warehouse on Cloud already have significant levels of automation. IBM’s vision is to bring full automation to the Cloud Pak for Data platform, building on a data fabric foundation.
Cautions
Fragmented Sales and Marketing: While IBM’s vision is now unified behind Cloud Pak for Data, the sales and marketing teams are still fragmented so it may be hard to obtain information. IBM has a massive portfolio of product offerings, and different teams may still have a specific focus that is not outwardly aligned with the core vision.
Fully Managed PaaS With Still Limited Availability: Cloud Pak for Data is built on OpenShift containerized infrastructure. Depending on where it is deployed, customers will need to manage more components of the stack. Fully managed “as a service” operations are currently only available in IBM Cloud, or via IBM Cloud Satellite for selected offerings.
Inconsistent Experience With Implementations: Users of Gartner’s client inquiry service have reported issues with implementations of Cloud Pak for Data when deploying on non-IBM public cloud infrastructure. These issues are very likely transient and should resolve as the volume of implementations increases.
InterSystems
InterSystems is a Challenger in this Magic Quadrant. It sells InterSystems IRIS, a multi-model, hybrid DBMS supporting both relational and nonrelational data models for transactional and analytics workloads. InterSystems also has many customers still using its InterSystems Cache product.
InterSystems’ operations are geographically diversified. Its clients tend to be of all sizes. They come primarily from the healthcare sector, but the vendor also has a presence in other industries, such as manufacturing, financial services, government and retail. InterSystems IRIS is currently available as a public, fully managed dbPaaS on AWS, GCP, Microsoft Azure and Tencent. A private, fully managed dbPaaS version is also available.
Strengths
Proven Vendor, Reliable Offering: InterSystems has a long history in the healthcare industry, where it earned a reputation for reliability, scalability and interoperability. InterSystems IRIS has demonstrated these attributes both as a stand-alone product and a platform for many offerings from ISVs. As InterSystems has broadened its reach into more industries and cloud environments, the product’s historical strengths have become the primary reason for the vendor’s growth.
Loyal Customer Base: Judging from discussions with users of Gartner’s client inquiry service, existing InterSystems IRIS customers want to use InterSystems for additional systems, mainly due to the support and functionality it offers. Customers have acquired InterSystems IRIS as a platform for purchased applications and now want to extend its use to analytics tools.
Multi-cloud, Hybrid and Intercloud: Given InterSystems IRIS’s on-premises basis, it becomes a hybrid alternative as cloud adoption grows. InterSystems IRIS is also multi-cloud (it supports the major CSPs) and intercloud (a single SQL query run by InterSystems IRIS can access multiple cloud DBMSs and object stores).
Cautions
Lateness to Market: InterSystems was late to offer a managed dbPaaS on public and private clouds. This may not have hurt it in the healthcare industry, which has demonstrated general reluctance to embrace the cloud, but its lateness has put InterSystems behind its competitors in the cloud DBMS market.
Market Competition: As InterSystems moves into the fully managed, multi-cloud dbPaaS market, it will face even more competition than it did with on-premises, as it will be competing with native cloud CSPs and ISVs. Customers will struggle to understand the competitive differentiators.
Limited Availability of Skills: Outside the healthcare sector, InterSystems is not commonly known in the broader cloud DBMS market. So, customers may struggle to find personnel skilled with InterSystems IRIS and other InterSystems products.
MariaDB
MariaDB is a Niche Player in this Magic Quadrant. It sells MariaDB SkySQL, a fully managed cloud offering of the MariaDB database that is also available as an on-premises offering in both MariaDB Enterprise and the fully open-source MariaDB Community Server as befits its long MySQL heritage.
MariaDB’s operations are primarily split between North America and Europe, with a limited presence in the Asia/Pacific region. Its customers span a wide range of industries and enterprise sizes. MariaDB’s capabilities were evaluated for both operational and analytics use cases for this Magic Quadrant.
Strengths
Unified Operational and Analytics: MariaDB provides multiple storage engines to support different types of workloads: row-store-oriented transaction processing with InnoDB, distributed SQL with Xpand and analytics processing using ColumnStore. MaxScale provides an SQL proxy to automatically route queries to the appropriate storage engine, supporting a full range of capabilities. Support for MongoDB API, PL/SQL and geospatial adds to the diversity of supported workloads.
Multicloud, Hybrid and Oracle Compatibility: MariaDB SkySQL is available in AWS and GCP. Support for Azure is imminent. All versions, both in the cloud and on-premises, offer compatibility and support for Oracle PL/SQL, making it easy to migrate from Oracle environments.
Financial Governance Vision: MariaDB has introduced a feature called Workload Analysis (WLA), which learns and predicts workload usage across a SkySQL cluster. Today, this provides instrumentation and observability, but it is the first step toward a broader vision on recommendations and automation for quality of service within cost constraints, leveraging ML-based predictive analytics.
Cautions
Strong Competition: As with any ISV, MariaDB faces strong competition from both native cloud service providers and more established ISVs. Every CSP has a MySQL or MySQL-compatible offering, many of which are deeply integrated into the CSP infrastructure.
Limited Commercial Market Presence: While MariaDB has become the de facto standard for MySQL-compatible DBMS offerings in the open-source community (including most Linux distributions), this has yet to manifest itself in commercial revenue in the broader market. MariaDB remains near the bottom of Gartner’s named vendors for DBMS tracked by revenue, with less than 1% market share in a nearly $65 billion market.
Cloud Offering Is Relatively New: MariaDB SkySQL is a relatively new offering and was only introduced to market in 2020. MariaDB will have to demonstrate broad adoption in the cloud if it is to be successful.
MarkLogic
MarkLogic is a Visionary in this Magic Quadrant. Its MarkLogic Data Hub Service is offered on the AWS and Azure clouds. MarkLogic focuses on data management, built around a transactional document store and an integration hub. The integration hub enables users to access data stored remotely through a universal index, which enables reduced remote data movement through optimization of remote access.
MarkLogic’s operations are primarily in North America and Europe. It has customers in a range of industries, but particularly in the public/government, finance and insurance, and healthcare sectors.
Strengths
Product Addresses Key Data Integration Need: MarkLogic has focused on implementing its data hub as a unique approach to data integration that allows it to include data in remote sources not only in its catalog, but also in the key index used to access data. This approach provides flexibility, as underlying data sources can be moved without interfering with access, as well as enabling improved performance by reducing the amount of remote data that has to be transferred.
Depth of Product Capabilities: MarkLogic’s platform has many capabilities that can help a range of use cases. They include fast ingestion, multi-model support, advanced security, graph support, and a very broad set of indexes and performance features that enable MarkLogic to address both operational and analytics use cases.
Pricing Model: The MarkLogic Data Hub Service has a unique pricing model that combines predictable cost with the ability to store excess credits for use for bursting requirements. This enables dynamic scalability with predictable pricing.
Cautions
Mind Share: MarkLogic continues to lag behind in reaching widespread market recognition or awareness. Although it has expanded the use cases it readily supports and has been good at expanding its footprint in existing accounts, its lack of mind share still hinders its overall growth.
Scarcity of Expertise: Potential customers of MarkLogic can struggle to find available resources to model with and help implement MarkLogic’s offering optimally. Given the depth of the vendor’s capabilities, this is seen as a limiting factor for widespread successful implementations.
Competition on Vision: The MarkLogic vision of being a universal hub for data access is very compelling — so compelling that many other vendors are looking to provide some of the same benefits. Since these vendors have more market awareness, this could slow adoption of MarkLogic, which would in turn slow awareness and expertise growth.
Microsoft
Microsoft is a Leader in this Magic Quadrant. It provides a broad range of cloud DBMS offerings, including Azure Synapse Analytics, Azure SQL Database, Azure SQL Managed Instance, Azure Cache for Redis, Azure SQL Edge, Azure Cosmos DB, Azure HDInsight, and Azure Database for PostgreSQL, MySQL and MariaDB.
Microsoft offers a comprehensive cloud data management ecosystem that spans all the use cases evaluated for this Magic Quadrant. Its operations are geographically diversified, and its customers cover a wide range of industries and deployment sizes.
Strengths
Comprehensive Data Ecosystem Vision: Microsoft has articulated a strong data ecosystem vision with Azure Synapse Analytics. This vision is more refined and comprehensive than that of most of its main CSP competitors, and it includes end-to-end security and metadata support. Easy integration with other Azure offerings (via Synapse Link and Purview) is a major selling point, and the ecosystem is open to third-party ISV offerings as well.
Clear Migration Path: Almost every enterprise has a business relationship with Microsoft, through the use of its data management products (such as Microsoft SQL Server), office productivity tools, identity and access management software, and other offerings. Azure represents a natural extension of this familiar Microsoft ecosystem and a low-risk migration path to cloud, with a comprehensive set of cloud offerings and a strong user community with relevant skills.
Integrated Multi-model Capabilities: Unlike some of its CSP competitors, Microsoft has embraced a multi-model strategy for many of its data management offerings, which can simplify deployment. Azure Synapse reflects this strategy for analytics, and Azure Cosmos DB epitomizes it for nonrelational operational DBMSs. The two can be used together for high-performance augmented transactions, with minimal data movement or redundancy.
Cautions
Inconsistent Ecosystem Maturity: Some key components of Microsoft’s cloud ecosystem are still under construction — namely, Purview, Synapse Link (for Dataverse) and industry data models. Prospective users should carefully check that Microsoft’s current capabilities meet their needs, and that timelines for the delivery of additional required functionality are clear.
Pricing and Cost Challenges: Microsoft’s overall pricing model is complex and challenging, resulting in a high volume of client inquiry to Gartner’s Software Pricing and Vendor Management team. Users must carefully assess the costs of Azure offerings within that context.
Data Architecture Challenges: Although Microsoft’s portfolio of cloud and on-premises DBMSs is comprehensive, it is inconsistent in that deployment options for public cloud, private cloud and traditional on-premises vary on a per-product basis. On the upside, this enables hybrid architectures for users that need them. On the downside, users must decide carefully where they will manage specific datasets and run diverse data processing workloads.
Oracle
Oracle is a Leader in this Magic Quadrant. Oracle Autonomous Database (including the Autonomous Transaction Processing and Autonomous Data Warehouse services) are available in Oracle Cloud Infrastructure (OCI) and on the Oracle Exadata Cloud@Customer (ExaCC) private cloud. Oracle also offers Autonomous JSON Database, Oracle MySQL Database Service, Oracle NoSQL Database and Oracle Big Data Service. Oracle Dedicated Region Cloud@Customer private cloud runs all Oracle cloud services in the customer’s data center.
Oracle’s operations are geographically diversified. The vendor has clients from all industries and of all sizes.
Strengths
Augmented DBMS Technology: The Oracle Autonomous Database uses AI- and ML-assisted tuning and leverages Oracle’s cloud infrastructure, including zero-downtime patching and upgrading. This technology not only reduces the necessity for manual operation, but also reduces cost and increases performance of the DBMS service.
Hybrid Cloud: Oracle has a complete hybrid environment for sharing data and enabling migrations to the cloud. It supports on-premises deployments and ExaCC with disaster recovery in the cloud via Exadata Cloud Service (ExaCS). ExaCC supports both Autonomous Data Warehouse and Autonomous Transaction Processing.
Richness of Portfolio: Oracle Cloud is a good choice for enterprises that use Oracle as a standard provider, where they can apply skills developed over many years to use cases in the cloud. With Oracle Autonomous Database’s free tier being adopted by customers new to Oracle that value a self-driving enterprise DBMS, Oracle is gaining recognition as an enterprise cloud provider.
Cautions
OCI-Centric DBMS Strategy: Only Oracle’s own DBMS services have been available as managed services on OCI; Oracle will offer a PostgreSQL managed service in 2022. While Oracle Database is generally portable to other clouds, Oracle Database clients will pay for twice as many virtual CPUs when running on other clouds. Oracle Real Application Clusters (RAC) is not supported on other clouds, and Oracle Database is not yet certified to run on all major CSPs.
Premium Price Perception: Oracle’s on-premises products are often perceived to be expensive and difficult to manage, and customers continue to raise concerns about contract negotiations. Oracle has made progress in these areas as it moves to a new business model on the cloud with pay-as-you-go pricing, the self-driving Autonomous Database, an Always Free tier, bring your own license (BYOL) and dynamic elasticity. In addition, free Oracle Cloud Lift Services will help customers migrate to OCI, and a Support Rewards program offers rewards for spending on OCI services. Customers should carefully evaluate proposals to maximize value.
Cloud Transition Focus: Oracle was very slow to offer a true dbPaaS managed service. This has caused enterprises to use other dbPaaS offerings from CSPs and ISVs. According to Gartner’s numbers, Oracle’s share of the dbPaaS market for 2020 ($875.9 million out of $26.2 billion) was flat at 3.3%. Non-Oracle customers should carefully evaluate near-term cloud migration choices.
Redis
Redis is a Challenger in this Magic Quadrant. It offers the Redis Enterprise Cloud on the AWS, GCP and Azure clouds, while Redis Enterprise Software is available for on-premises deployments. Its offerings are built on the open-source Redis product. Redis’ operations are primarily in North America, EMEA and the Asia/Pacific region, but are also strong in Latin America. It has a limited presence in the rest of the world. Redis has customers — both large and small — in a broad range of industries.
Redis Enterprise Cloud is an in-memory, nonrelational, multi-model data store, which includes a range of capabilities suited to operational DBMS use cases and augmented transaction processing. Its flexible data structures and integrated support for transaction augmentation via RedisGears and RedisAI are particularly noteworthy. It is a good potential replacement for a pure data grid.
Strengths
Extreme High Performance: The in-memory nature of Redis Enterprise Cloud accounts for the extreme high performance that Redis is known for (especially in transaction processing), and the vendor guarantees sub-millisecond responses for some configurations and operations.
AI/ML Use Cases: Redis caters well to the growing areas of AI and ML by providing RedisAI (a very high-performance inferencing engine), an online feature store and streaming data processing.
Multi-model and Multiplatform: Redis supports multiple data models, including native JSON, graph and time series data. By working with Redis data types, developers can create almost any model. It runs on multiple cloud platforms, on-premises, and in hybrid and distributed configurations.
Cautions
In-Memory Data Cache Perception: Redis’ success with its in-memory data cache has made it a successful vendor, but frequently either as a supplement to an incumbent DBMS vendor or as a result of demand from application developers. This limits Redis’ perceived strength and its overall growth opportunities in the DBMS market.
Eventual Consistency: To protect high performance, Redis did not enable implementation of strong consistency; however, this has recently been rectified. This is a relatively new feature and users should verify that it is suitable for their transactional workloads.
Limited Data Warehouse Usage: Redis offers features that can be useful in data warehousing, namely RedisTimeSeries, RedisGraph and RedisConnect. However, users should note that Redis’ limited support for SQL and the rest of the relational paradigm limits its applicability to data warehousing.
SAP
SAP is a Leader in this Magic Quadrant. Its products include SAP HANA Cloud, SAP Data Warehouse Cloud, SAP Adaptive Server Enterprise, SAP IQ and SAP SQL Anywhere. Products address both operational and analytical DBMS use cases. SAP operates on a global basis from diverse locations. It has enterprise customers of all sizes from all industries. For analytical use cases, the vendor offers SAP Data Warehouse Cloud for SQL-based development of data warehouses for both SAP and non-SAP data, and SAP BW/4HANA, a packaged data warehouse application that can be deployed on-premises and in the cloud.
SAP HANA is available on multiple public clouds as a fully managed service, an appliance, on a virtual machine and as software only.
Strengths
Breadth and Depth of Functionality: SAP HANA-based systems provide unified analytical and transactional processing against a single copy of data, embedded predictive analytics and multi-model capability with embedded graph features, language processing, OLAP engines, data virtualization, and Hadoop, Spark and cloud object stores.
Track Record: SAP HANA is widely accepted by SAP customers, with steady adoption for SAP S/4HANA and SAP BW/4HANA, other applications, and as a general-purpose analytical system.
Multi-cloud, Intercloud and Hybrid: SAP HANA can be hosted on a wide variety of platforms, including the Alibaba, AWS, Azure and GCP public clouds, and SAP Business Technology Platform. SAP HANA can also be deployed as an appliance, using hardware from a variety of vendors, on hyperconverged systems, bare-metal installations and virtual machines.
Cautions
Marketing and Sales Messaging: Many SAP clients still tell Gartner that they are unaware of the breadth and depth of SAP’s data management offerings. SAP customers should ensure that they are being fully informed about the vendor’s offerings in this space. SAP customers should represent a good number of low-hanging fruit.
Mainly SAP Customers: Despite offering a general-purpose suite of data management tools, there appears to be limited adoption by organizations not already using SAP. It appears that SAP sales does not evangelize its data management outside of its large customer base. Organizations should include SAP on their lists for consideration as a major data management vendor, even if they are not current SAP application customers.
Cost Estimates: Incorrect sizing and configuration, where hot and cold data schemes and compression factors have not been properly accounted for, can result in the perception of higher costs. Customers should ensure that they understand the basis of any pricing — the more transparent cloud-based pricing should assist with this.
SingleStore
SingleStore is a Niche Player in this Magic Quadrant. A new entrant, it offers SingleStore Managed Service, a fully managed, on-demand cloud database service that is compatible with the MySQL wire protocol. It can be deployed on the AWS, GCP and Microsoft Azure clouds, as well as on private clouds, IBM Cloud Pak for Data and Red Hat OpenShift. SingleStore’s operations are primarily in North America and Europe. It has a limited presence in the rest of the world. It has customers — from small to large — in information management, technical services, finance and insurance, as well as other industries.
SingleStore provides a Universal Storage technology, which combines the attributes of in-memory row store, on-disk column store and cloud object storage to support high-performance use cases for both transactions and analytics.
Strengths
Integrated Transactions and Analytics: SingleStore’s high performance applies to both transactions and analytics (including queries and analytics models). In fact, these workloads and others can execute concurrently and still achieve high performance for mixed use cases, such as augmented transactions and real-time operational analytics. SingleStore Managed Service has a multi-model data store and a cloud-native serverless architecture that unifies column store, row store, geospatial data types, JSON, full text, key value and time series.
High Performance: SingleStore was built to achieve large transaction counts, even with complex transactional workloads, as seen in augmented transactions. SingleStore is also known for high-performance database operations, such as complex joins, multicolumnar queries, thousands of concurrent users and balancing multiple APIs.
Strong “Data in Motion”: SingleStore pipelining enables continuous, parallel loading without data locking or performance overhead. High-speed windowing and time series updates enable background bulk loads, operational analytics and monitoring via data streams.
Cautions
Limited Language Support: SingleStore lacks onboard support in the DBMS for Java, Python, Graph and other languages that some data scientists or data engineers require when programming their own algorithms for in-database analytics or other in-DBMS processing. As a workaround, users can call user-defined programs, written in any language, via a MySQL-compatible wire protocol.
Limited SQL Support: SingleStore customers that have experience with other relational platforms have commented that SingleStore’s SQL implementation has some limitations. For example, SingleStore does not support triggers, foreign keys and referential integrity, and prepared statements.
Limited Native Monitoring: SingleStore monitoring is immature but will improve. In the meantime, users must deploy third-party tools or write scripts that process system information for DBMS monitoring purposes.
Snowflake
Snowflake is a Leader in this Magic Quadrant. The Snowflake Data Cloud, available on AWS, GCP and Microsoft Azure, is focused on delivering a global, integrated analytics-focused data environment for data warehouse as a service, data lake and data marketplace delivery. The platform also supports document-style data (such as JSON), data science and a private cloud offering called Virtual Private Snowflake, although this is not an on-premises option.
Snowflake operates mostly in North America, but continues to gain traction in EMEA and the Asia/Pacific region. Its customers cover a wide range of industries and deployment sizes. Snowflake was evaluated for its analytics use-case capabilities for this Magic Quadrant.
Strengths
Strong Market Presence and Robust Partner Ecosystem: Snowflake is an integral part of the cloud data warehouse market landscape, with very high customer and partner satisfaction, market-leading revenue percentage growth, and consistently high interest among Gartner clients. Robust market traction with system integrators and partners in data science, data integration, BI and other areas solidifies its market presence. Its growth accelerated in 2020.
Operational Ease of Use and Scalability: Snowflake’s core design principles focus on operational ease of use and scalability. Dynamic resource allocation in response to changing workloads remains best in class. Ease of use and reliability are frequently praised in Gartner Peer Insights.
Data Sharing and Data Marketplaces: Snowflake has popularized the concept of data sharing, collaboration and data marketplaces, providing a platform enabling easy monetization of proprietary datasets. A network effect is driving significant traction and adoption of the platform as a result.
Cautions
Financial Governance Remains a Challenge: Gartner clients and reviewers on Gartner Peer Insights report challenges with financial governance and cost control. A new financial governance dashboard, released in the second quarter of 2021, should help clients with budget planning, cost allocation and predictability. Additionally, core services such as governance and metadata may incur additional costs if usage exceeds certain thresholds, although Snowflake states that this is rare.
Changing Competitive Arena: When Snowflake first launched its offering six years ago, it was highly differentiated. Today, its primary competitors are increasingly capable and Snowflake’s differentiation has narrowed in some areas, while continuing to progress in others. Clients should ensure that Snowflake’s offerings meet their functional and price/performance requirements when compared to competitors.
Platform Openness: Snowflake’s vision for an intercloud data cloud allowing customers to access data wherever it may lie is appealing, but is not yet fully proven. Snowflake external tables support external data access via open standards and we expect the vendor to continue to add support for additional open data standards in the future.
Teradata
Teradata is a Leader in this Magic Quadrant. It focuses on analytical use cases. Teradata Vantage provides a unified set of analytics capabilities, such as graph processing, ML and text processing in the Teradata database.
Teradata’s operations are geographically diversified. Its customers tend to be large and from all industries. Teradata continues to invest in its hybrid multi-cloud ecosystem, which integrates many different styles of database designs and analytics on a common platform. Teradata Vantage is delivered in a consistent manner across multiple public clouds, Teradata’s own cloud service and on-premises.
Strengths
Positioned for Data Fabric: Teradata has many years of experience using its DBMS and QueryGrid technology to bring to bear on data fabric requirements. This includes mature distributed data access, advanced optimizer technology and robust workload management.
Multi-cloud/Intercloud/Hybrid Deployment: Teradata combines SQL, ML, graph, multi-model and federation in an easy-to-use system that can be deployed in multiple public and private clouds plus hybrid architectures, including on-premises.
System and Financial Predictability: Teradata Vantage provides operational reliability and high throughput. Price predictability and financial governance are key strengths. Customers praise Teradata for its ability to support very large complex workloads and large concurrent user bases. Teradata performs well in terms of operational intelligence, a critical capability for cloud DBMS use cases.
Cautions
Focus on Analytical Use Cases: Teradata does not offer a general-purpose or operational database, although it does perform well in terms of operational intelligence. It should be considered by organizations wanting a best-of-breed analytical cloud DBMS.
Legacy Positioning: Teradata was incorporated in 1979 and, together with other long-lived data warehouse vendors, may be dismissed from consideration by being misclassified as “old technology.” Organizations should judge these vendors on their merits.
Cost Perception: Teradata has been perceived as a high-cost, “premium” option. However, its move to the cloud and its new “pay for what you use” pricing model, together with the transparency of cloud billing, should enable it to make its cost-to-performance benefits more apparent. Potential customers should request a proof of concept to properly understand Teradata’s price/performance.
Vendors Added and Dropped
We review and adjust our inclusion criteria for Magic Quadrants as markets change. As a result of these adjustments, the mix of vendors in any Magic Quadrant may change over time. A vendor's appearance in a Magic Quadrant one year and not the next does not necessarily indicate that we have changed our opinion of that vendor. It may be a reflection of a change in the market and, therefore, changed evaluation criteria, or of a change of focus by that vendor.
Added
Cockroach Labs
Couchbase
Exasol
MariaDB
SingleStore
Dropped
Tencent was dropped because it did not meet Gartner’s market momentum threshold. Please note that this is not a reflection on the vendor’s products, but more likely due to its heavy focus on China.
Inclusion and Exclusion Criteria
Gartner Magic Quadrants identify and analyze the most relevant providers in a market. Gartner imposes an upper limit of 20 vendors to aid in identification of the most relevant providers. The following inclusion criteria represent the specific attributes that Gartner analysts consider necessary for a vendor to be included in this Magic Quadrant.
Inclusion Criteria
To qualify for inclusion in this Magic Quadrant, a vendor had to:
Offer a generally available software product that met Gartner’s definition of a cloud DBMS.
Support at least three of the following cloud DBMS use cases:
Traditional transactions
Traditional data warehouse
Logical data warehouse
Augmented transaction processing
Event stream processing (Internet of Things)
Data science exploration/deep learning
Operational intelligence
Rank among the top 20 organizations in a market momentum index defined by Gartner for this Magic Quadrant. Data inputs used to calculate market momentum include the following measures, among others:
Gartner customer search and inquiry volume and trend data
Volume of job listings on a range of employment websites in the U.S., Europe and China
Frequency of mentions as a competitor to other cloud DBMS vendors in reviews on Gartner Peer Insights during the year ending March 2021
Have market presence in at least three of the following regions (regional market presence is defined as a minimum of 5% of the revenue of the verified production customer base, as well as the existence of dedicated sales offices or distribution partnerships in a specific region):
North America (Canada, Mexico and the U.S.)
Central and South America
Europe (including Western Europe and Eastern Europe)
Middle East and Africa (including North Africa)
The Asia/Pacific region
Japan
Have a cloud DBMS service generally available as of midnight, U.S. Eastern Daylight Time on 1 July 2021. This included any new functionality added to the service by the specified date. We did not consider beta, “early access,” “technology preview” or other not generally available functionality or services. Additionally:
Any acquired product or service must have been acquired and offered by the acquiring vendor as of 1 July 2021. Acquisitions after this date were considered under their preacquisition identities, if appropriate, and are represented separately until the publication of the following year’s Magic Quadrant.
Exclusion Criteria
Vendors marketing only products from the following list are explicitly excluded from this Magic Quadrant and its companion Critical Capabilities research:
“Streaming” services, which have use cases that are dominated by immediate event processing and which are rarely, if ever, used for subsequent management of the data involved
Pre-relational DBMS products
Object-oriented DBMS products
Data grid products
BI and analytical solutions that offer a cloud DBMS that is limited specifically to the vendor’s own BI and analytical tools
Analytics query accelerators (SQL interfaces to object stores or file systems)
Vendors of data virtualization, data fabric and data federation that do not provide data persistence of their own
Honorable Mentions
The vendors mentioned below were either featured in the 2020 edition of this Magic Quadrant or have momentum that may make them of interest to organizations looking beyond the vendors covered in this year’s Magic Quadrant. The following list, which does not include all the notable vendors absent from this Magic Quadrant, is in alphabetical order:
Crate.io — Crate.io offers CrateDB, an enterprise-grade open-source database, enabling data insights at scale. It is the building block for data-intense analytics and AI solutions. CrateDB’s ability to ingest and manage massive amounts of data from diverse sources empowers companies worldwide to turn data into business value. It aims to combine the familiarity of SQL with the scalability and data flexibility of NoSQL. This enables developers to use SQL to process any type of data — structured or unstructured — and to perform SQL queries at real-time speed. CrateDB is designed to be easy to scale as an organization grows in the cloud, at the edge and on-premises. Optimized for large data volumes with high compute and data versatility requirements, CrateDB has ingestion speeds of millions of data points per second. Crate.io did not appear in this year’s Magic Quadrant because it did not score in the top 20 of Gartner’s market momentum index.
DataStax — DataStax Astra DB, which is available on AWS, GCP and Azure, is the vendor’s multi-model, multi-cloud, multi-region, serverless, pay-as-you-go managed service built on Apache Cassandra. DataStax also offers DataStax Enterprise, a multi-model DBMS based on Apache Cassandra, for enterprises that want to self-manage their databases. It supports wide column, key value, document and graph models, as well as streaming analytics. Astra Streaming, based on Apache Pulsar, provides real-time event stream-processing and change data capture (CDC) capabilities to connect a broad range of DBMS offerings to Apache Cassandra with bidirectional CDC. DataStax declined to participate in this Magic Quadrant as it was still ramping up its cloud offering, DataStax Astra, during Gartner’s evaluation period.
EDB (formerly EnterpriseDB) — EDB’s PostgreSQL managed cloud database service, BigAnimal, is a relational, multi-model, multi-cloud platform based on open-source PostgreSQL with Oracle compatibility. It uses a Kubernetes-based control plane for automating the full database life cycle. EDB was purchased by Great Hill Partners in 2019 and is now privately held. It does not appear in this year’s Magic Quadrant because, at the time of this research, it did not offer a managed cloud service.
Micro Focus — Vertica is a massively parallel processing (MPP) column store analytical database that can run in the Alibaba, AWS, Azure or GCP public clouds as Kubernetes containers, on virtual machines and on-premises including a separation of compute and storage. It is compatible with many modern extraction, transformation and loading (ETL) and BI tools. The Vertica Unified Analytics Platform combines data in the data warehouse and data lake for SQL, ML and AI. Vertica did not appear in this year’s Magic Quadrant because, at the time of this research, Vertica Accelerator, a fully managed service on AWS, was available only in beta for select customers. It is now commercially available as a fully managed service globally.
MongoDB — This is a multi-model DBMS, based on a document store, with time series and search engine capabilities. MongoDB Atlas is a managed cloud offering available on AWS, Azure and GCP. Its market performance is outstanding, and it has been one of the most successful vendors in moving to the cloud. This vendor did not respond to requests to participate in this year’s Magic Quadrant. This is the fifth consecutive year of nonparticipation for MongoDB, thus our information on the vendor’s strategy and roadmap is significantly outdated. As a result, we have not attempted to assess MongoDB in this Magic Quadrant.
Neo4j — Headquartered in San Mateo, California, Neo4j and has offices in Sweden, Germany, Singapore and the U.K. It specializes in graph database and graph data science technology. The Neo4j graph database is ACID-compliant and designed for enterprise environments, with native graph storage and a distributed cluster architecture, as well as a rich collection of code-first and code-free tooling, drivers and frameworks aimed at OLTP use cases. The Neo4j Graph Data Science Library provides integrated AI/ML capabilities and workflows specifically targeted at data scientists and their analytical pipelines. It is available in as-a-service (Neo4j AuraDB on AWS and GCP, and AuraDS for data scientists — early access as of date of publication of this Magic Quadrant), as a managed service and in self-hosted deployment models. Graph databases are a subset of the wider transactional and analytics DBMS marketplace but can be appropriate for the right graph-oriented use cases. Neo4j did not appear in this year’s Magic Quadrant because it did not score in the top 20 of Gartner’s market momentum index.
Tencent — Tencent Cloud offers a full range of cloud database management services, including operational and analytical database services, as well as a wide range of other cloud-based services. Its products support implementation in the public cloud, private cloud (Tencent Cloud Enterprise [TCE]) and hybrid cloud. Tencent’s operations are primarily in the Asia/Pacific region, and it is also expanding business in Europe, North America and South America. It has hundreds of regional and industry channel partners that remarket its products. More than 30 industries are represented in its user base, which includes companies of all sizes. Tencent did not appear in this year’s Magic Quadrant because it did not score in the top 20 of Gartner’s market momentum index. Operational database services include: cloud relational database TencentDB (for MySQL, MariaDB, PostgreSQL and SQL Server); enterprise distributed DBMS TDSQL (for MySQL and PostgreSQL); cloud-native database TDSQL-C (for MySQL and PostgreSQL); TencentDB (for Redis and MongoDB); TencentDB for TcaplusDB, a distributed NoSQL DBMS for games; Tendis, a key value storage NoSQL DBMS; and time series database CTSDB. Analytical database services include: cloud data warehouse (for PostgreSQL and ClickHouse); Oceanus, a real-time big data analysis platform based on Apache Flink; Elastic MapReduce (EMR), an elastic cloud-native Hadoop service; Tencent Big Data Suite (TBDS); TDSQL (for MySQL, PostgreSQL); and Tencent DB for Graph Database (KonisGraph). Tencent Cloud also provides TDSQL-H for HTAP use cases, as well as a full range of database SaaS tools, such as database autonomous service DBbrain, Data Transmission Service (DTS), Database Expert Service (DBexpert) and Tencent Cloud Elasticsearch Service (ES). Tencent did not appear in this year’s Magic Quadrant because it did not score in the top 20 of Gartner’s market momentum index.
TigerGraph — TigerGraph provides a graph AI platform that is built on a distributed native graph database that comes with an SQL-like query language and tightly integrates tooling and enterprise connectors to ensure that data scientists and developers can design and deploy analytical solutions within weeks. TigerGraphDB is a native, distributed MPP graph database that supports AI and data science practices. It stores data as entities and the relationships between those entities to enhanced decisions using combinations of prescriptive and predictive analytics, in graph database ML, and enables faster discovery of insights by connecting internal and external data. This aims to provide faster delivery of an advanced infrastructure that more ably supports enhanced visualization and display capabilities. Industry solutions are also offered. The vendor offers Integrated user experience (UX) toolkits developed for industry-specific use cases, such as Digital Twin, Data Operations Impact Analysis, Fraud/AML and C360. TigerGraph did not appear in this year’s Magic Quadrant because it did not score in the top 20 of Gartner’s market momentum index.
Yellowbrick Data — Yellowbrick Data provides a data warehouse solution for hybrid environments that features novel cloud infrastructure exploitation to provide extreme performance and ease of use for classical data warehouse analytical requirements. In addition, the vendor is well-positioned for data fabric adoption through what it terms “distributed clouds,” interconnected resources across private data centers, multiple public clouds and on-premises. Its system is PostgreSQL-compatible and thus integrates well with common BI, analysis and ETL tools. In addition, it addresses high-volume, high-speed requirements such as IoT and deployment at the network edge. It provides for modern requirements such as integration with data lakes. Yellowbrick Data did not achieve a top 20 ranking in Gartner’s market momentum index, which was required for full coverage in this Magic Quadrant.
YugabyteDB — This is a distributed SQL database that provides the horizontal scalability of NoSQL systems and the ACID guarantees and relational modeling of traditional SQL systems. YugabyteDB is wire- and code-compatible with PostgreSQL. Critical services can remain available during node, zone, region and data center failures with fast failovers. It delivers zero downtime on maintenance tasks such as software upgrades, security patching and distributed backups, and can scale out even under heavy load without disruption or downtime by simply adding nodes to the YugabyteDB cluster. The database automatically rebalances load, and its shared-nothing architecture is designed with no single point of failure or bottleneck. YugabyteDB offers comprehensive and flexible deployment options in geo-distributed environments. Operators can make use of synchronous and asynchronous data replication and geo-partitioning to achieve desired consistency, resilience, performance and compliance objectives. Yugabyte did not appear in this year’s Magic Quadrant because it did not score in the top 20 of Gartner’s market momentum index.
Evaluation Criteria
Ability to Execute
Product or Service: Core goods and services that compete in and/or serve the defined market. This includes current product and service capabilities, quality, feature sets, skills, etc. We look at products and services that address both operational and analytics use cases. We focus on features influencing performance, scalability, availability, security and integration.
Overall Viability: Includes an assessment of the organization’s overall financial health as well as the financial and practical success of the business unit. We assess the likelihood of the organization to continue to offer and invest in the product, as well as the product position in the current portfolio. We use Gartner published estimates on revenue, as well as our assessment of share of market and trends in revenue. Changes in organization structure, personnel, and roadmap are also included.
Sales Execution/Pricing: The organization’s capabilities in all presales activities and the structure that supports them. This includes deal management, pricing and negotiation, presales support and the overall effectiveness of the sales channel. We also evaluate the variety and suitability of a vendor’s pricing models.
Market Responsiveness/Record: Ability to respond, change direction, be flexible and achieve competitive success as opportunities develop, competitors act, customer needs evolve and market dynamics change. This criterion also considers the vendor’s history of responsiveness to changing market demands. Timely creation of and fielding of a cloud offering, as well as competitive feature development and delivery in “cloud release cadence,” will be considered.
Marketing Execution: The clarity, quality, creativity and efficacy of programs designed to deliver the organization’s message in order to influence the market, promote the brand, increase awareness of products and establish a positive identification in the minds of customers. This mind share can be driven by a combination of publicity, promotional activity, thought leadership, social media, referrals and sales activities. We will compare the consistency, channels, volume and differentiation of marketing messages heard by prospects to those presented to analysts.
Customer Experience: Products and services and/or programs that enable customers to achieve anticipated results with the products evaluated. Specifically, this includes quality supplier/buyer interactions, technical support or account support. This may also include ancillary tools, customer support programs, availability of user groups, service-level agreements, etc.
Operations: The ability of the organization to meet goals and commitments. Factors include quality of the organizational structure, skills, experiences, programs, systems and other vehicles that enable the organization to operate effectively and efficiently.
Table 1: Ability to Execute Evaluation Criteria
Evaluation Criteria | Weighting |
Product or Service | High |
Overall viability | Medium |
Sales Execution/Pricing | Medium |
Market Responsiveness/Record | Medium |
Marketing Execution | Medium |
Customer Experience | Medium |
Operations | Medium |
Source: Gartner (December 2021)
Completeness of Vision
Market Understanding: Concerns the ability to understand customers’ needs and to translate that understanding into products and services. Vendors that show a clear vision of their market listen to, and understand, customers’ demands, and can shape or enhance the market with their vision. We look for awareness of customers’ concerns about issues such as price transparency, license portability, migration assessment, execution and cost, security gaps, and intercloud and hybrid operations.
Marketing Strategy: Looks for clear, differentiated messaging consistently communicated internally, and externalized through social media, advertising, customer programs and positioning statements. We assess the clarity and consistency of messages that articulate the value of the cloud DBMS, especially as compared with similar on-premises products (where they exist) and other cloud DBMS offerings.
Sales Strategy: Looks for a sound strategy for selling that uses appropriate networks, including direct and indirect sales, marketing, service and communication. It also considers any partners that extend the scope and depth of a vendor’s market reach, expertise, technologies, services and customer base. We evaluate relationships between CSPs, ISVs and system integrators, use of app stores and co-marketing, and the degree of focus on the ease of onboarding.
Offering (Product) Strategy: An approach to product development and delivery that emphasizes market differentiation, functionality, methodology and features as they map to current and future requirements. We will be evaluating whether the product strategy builds on existing product strengths and moves toward addressing emerging market needs. This includes the response to such issues as separation of compute and storage, CSP-managed instance definitions and availability, and response to security requirements for object storage.
Business Model: Considers the design, logic and execution of an organization’s business proposition to achieve continued success. We consider the vendor’s relationships to CSPs, financial model changes, geographic and vertical adjustments driven by cloud opportunities, and the design of engineering and support in relation to cloud realities.
Vertical/Industry Strategy: The strategy to direct resources (sales, product, development), skills and products to meet the specific needs of individual market segments, including verticals. We look at breadth and depth of vertical and industry focus. Information on specific successes in particular verticals or industries is useful.
Innovation: Considers direct, related, complementary and synergistic layouts of resources, expertise or capital for investment, consolidation, defensive or preemptive purposes. We judge a vendor’s innovativeness by the extent of its truly new capabilities or combinations in the market, rather than by its matching of the capabilities of existing products from other vendors.
Geographic Strategy: Considers a vendor’s strategy to direct resources, skills and offerings to meet the specific needs of geographies outside its “home” or native geography, either directly or through partners, channels and subsidiaries, as appropriate for the geography and market. We examine factors such as local language support, on-the-ground resources and the marketing focus on multiple geographies.
Table 2: Completeness of Vision Evaluation Criteria
Evaluation Criteria | Weighting |
Market Understanding | High |
Marketing Strategy | High |
Sales Strategy | High |
Offering (Product) Strategy | High |
Business Model | Medium |
Vertical/Industry Strategy | Low |
Innovation | High |
Geographic Strategy | Medium |
Source: Gartner (December 2021)
Quadrant Descriptions
Leaders
Leaders generally demonstrate the most support for a broad range of DBMS use cases, based on support for a wide range of data types and diversity of deployment models (such as multi-cloud, intercloud and hybrid). These vendors demonstrate consistent customer satisfaction and strong customer support. Many have mature products created for the cloud or migrated to be cloud-native. Hence, Leaders generally represent the lowest risk for customers in the areas of performance, scalability, reliability and support. As the market’s demands change, Leaders demonstrate strong vision in support not only of the market’s current needs, but also of emerging trends. These include requirements for multi-cloud, intercloud and hybrid models, as well as native cloud serverless DBMS and financial governance with diverse pricing models. Finally, the marketing messages, product research and development, and delivery of Leaders suit today’s market for public and private cloud services.
Challengers
Challengers are stable vendors with strong, established offerings, but are somewhat lacking in vision for the cloud DBMS market. While it can be difficult for some vendors to improve both vision and execution at the same time, it is normal for some to have high scores for Ability to Execute one year and high scores for Completeness of Vision another year. Challengers normally show strong corporate viability and financial stability, and demonstrate strong customer support. However, they lack some features to support the latest trends in the cloud DBMS market, such as support for a broad set of use cases and a roadmap for moving to multicloud/intercloud implementations. Although they may be lacking in relation to some of the market’s innovative concepts, Challengers have strengths in relation to many of the Ability to Execute criteria.
Visionaries
Visionaries have a strong market understanding and a robust roadmap for the cloud DBMS market. They have innovative ideas about functionality and demonstrate advanced use of new deployment models. Typically, they have fewer customers and are smaller than Leaders. Although they may lack the market momentum of Leaders, they have potential for growth in the market, due, in many cases, to market-leading vision. Although Visionaries are often young and small vendors, they have innovative ideas that push the market — and Leaders — in new directions.
Niche Players
Niche Players generally deliver a highly specialized product with limited market appeal. They frequently do not support all cloud DBMS use cases, but often support fewer use cases well. Niche Players may lack one or all of the following: a strong or large customer base; the breadth of functionality of Leaders; penetration of a broad industry or geography; and proven, mature products that present low risk. However, if an organization has a need for the specific set of capabilities that a Niche Player provides, then it can be a good fit.
Context
This Magic Quadrant evaluates vendors that supply fully managed cloud DBMS services (dbPaaS offering) for some or all operational and analytical use cases. It should interest anyone involved in defining, purchasing, building or managing a cloud environment involving data management — notably, CIOs, CTOs, data and analytics leaders, infrastructure managers, database and application architects, database administrators, and IT purchasing managers. Database management systems are at the heart of digital business. Cloud-managed DBMS service revenue growth accounts for 93% of overall DBMS revenue growth. Most DBMS vendors with on-premises offerings have shifted their innovation and development efforts to managed cloud services. To gain a better understanding of this shift, see Market Share Analysis: Database Management Systems, Worldwide, 2020. The market continues to shift toward the cloud helping DBMS vendors to innovate, and allowing end-user organizations to move to the cloud or write new and innovative applications there. This Magic Quadrant assesses databases that run as cloud services. 2020 was the first year that Gartner combined the assessment of vendors offering analytical database systems (previously referred to as data management systems for analytics [DMSAs]) with the assessment of operational DBMSs. This was in line with the observation that the database market was converging, with vendors providing solutions for both types of DBMS — sometimes with the same product (see There Is Only One DBMS Market). In the Vendor Strengths and Cautions section above, we make clear whether the vendor addresses operational or analytical use cases, or both. While it is advantageous for a vendor to address both, it is not essential. A vendor can still be a Leader if it serves its single chosen market particularly well. This Magic Quadrant iteration also changed the inclusion criteria to remove the overall threshold for revenue and to instead use Gartner’s market momentum index, which gauges the global interest in a product. This has allowed a number of new entrants into the Magic Quadrant that will be relevant to Gartner clients; in particular, it permits the consideration of smaller, focused and innovative vendors. It is also important to remember that the scoring of the vendors in the Magic Quadrant is relative and not absolute. Movements of vendors from one iteration to another must be considered within this context.
Market Overview
This market continues to show growth in cloud revenue, growth in the percentage of revenue in the cloud versus overall DBMS revenue and the decreasing interest in on-premises products. This is according to Gartner’s client inquiry service.¹ Gartner’s DBMS market numbers2 and Gartner’s 2020 figures for the enterprise public cloud sector3 show overall DBMS market revenue of $64.8 billion, with $26.2 billion coming from cloud DBMS products (40% of the total DBMS market). More importantly, the cloud DBMS sector generated $8.8 billion of the $9.5 billion total for DBMS revenue growth (93% of total DBMS revenue growth). We forecast that, by 2022, cloud DBMS revenue will account for 50% of the total DBMS market revenue, bringing this prediction forward by one year from 2020. There is white space at the top and to the right of the Magic Quadrant this year, signaling that there is still room for improvement. The ability to offer multicloud, intercloud and hybrid is well-advanced, but not all vendors can do all three, nor do they do them equally well. Also, the handling of metadata by cloud DBMSs is becoming more important. Metadata is required to help discover data, and to understand its usage, governance, security, lineage and quality, plus as a basis for more-augmented data management capabilities, including optimization. It has become clear that metadata will not just be controlled by centralized specialized tooling, and DBMSs will participate more in the handling of metadata. This will assist with implementing data fabrics and data governance. Overall, other markets will leverage metadata in more innovative ways. This is an area that cloud DBMSs are expected to increasingly address. The initial move by vendors to the cloud is now nearly over, with developments now more about exploiting the cloud. The cloud-native vendors began by offering simple cloud offerings and are now expanding them by providing more-sophisticated features. Likewise, the traditional on-premises vendors have now, for the most part, successfully transitioned to the cloud. Where they aim to converge is with simple-to-use and reliable cloud services that are feature-rich. There is some recognition by CSPs that customers require interoperation among clouds, but this is not yet widespread nor required for all use cases and scenarios. For DBMS for analytics use cases, there is a high standard to meet. Effective SQL processing, integration with data lakes, and integration with AI and ML are now common. This makes it more difficult for new entrants to enter the market. Also, as CSPs fill out their feature set, it presents a challenge for ISVs to stay differentiated and thus sustain their growth. In the analytics space, the concept of data sharing has been popularized and multiple vendors have offerings, or have made announcements. While the concept of sharing data using a database is not in itself new, the new generation of data sharing features makes it easy for organizations to package up and sell data within a data marketplace, and for consumers rather than publishers to bear the cost of accessing the data and integrating it with their own data. The basic features are not hard for competitors to replicate, and we expect to see most large vendors provide them. However, it is not clear that user organizations will wish to adopt particular technology in order to access data, rather than subscribe to a more open-system-style approach — there is already at least one initiative of this type underway under the Apache Software Foundation. In addition, several vendors own important proprietary datasets, or have existing sets of customers that would constitute a natural marketplace. Multiple interconnected marketplaces are the likely direction. For operational DBMSs, traditional online transaction processing is well-served. Batch transaction processing has emerged as a use case for Spark, especially with the development of more-robust features within data lake software, such as those implementing the lakehouse. Another noticeable OPDBMS trend is the emergence of distributed database management systems (DDBMSs). At present, these are aimed at very special web-scale requirements, or for large geographic markets such as China. However, it may be that, over time, these will move to the mainstream, with more-localized systems simply having all the DDBMS components and data in one location — but with the ability to expand across multiple locations and clouds, or move to other locations, at will. However, for the time being, mainstream nondistributed DBMSs will continue to be able to address the needs of most organizations. The influence of open-source software APIs continues to grow. Most cloud vendors provide services for popular open-source database management systems (OSDBMSs), notably PostgreSQL and MySQL. More interestingly, several vendors — both CSPs and independent — provide DBMSs that have PostgreSQL- or MySQL-compatible APIs, but their own highly scalable back ends. Some of these also aim to provide a mix of transactional and analytical processing. The trend toward data ecosystems continues. A data ecosystem occurs where a provider competes not just on a point solution for a particular service (for example, data warehouse, data lake or ML), but instead on the way in which multiple services are integrated, making it easy for them to be used together. This trend is likely to grow, although, at the same time, some vendors will continue to compete on the basis of a best-of-breed solution (see The Impacts of Emerging Cloud Data Ecosystems: An Architectural Perspective). Finally, it is worth noting that, besides the large organizations offering many services, there are a number of vendors that focus on providing a particular set of functionality and only that functionality, and doing it well. Some of these vendors have been providing solutions in their market for many years, or may be innovators in their space. For end-user organizations that wish to acquire the specific capabilities they provide, they can be a good choice — what matters is the fit of the vendor and its offering to the actual requirements of the user organization.
Evidence
¹ Gartner client inquiry service data recorded from June 2020 through June 2021. ² Market Share: All Software Markets, Worldwide, 2020. ³ Market Share: Enterprise Public Cloud Services, Worldwide, 2020.
Evaluation Criteria Definitions
Ability to Execute
Product/Service: Core goods and services offered by the vendor for the defined market. This includes current product/service capabilities, quality, feature sets, skills and so on, whether offered natively or through OEM agreements/partnerships as defined in the market definition and detailed in the subcriteria. Overall Viability: Viability includes an assessment of the overall organization's financial health, the financial and practical success of the business unit, and the likelihood that the individual business unit will continue investing in the product, will continue offering the product and will advance the state of the art within the organization's portfolio of products. Sales Execution/Pricing: The vendor's capabilities in all presales activities and the structure that supports them. This includes deal management, pricing and negotiation, presales support, and the overall effectiveness of the sales channel. Market Responsiveness/Record: Ability to respond, change direction, be flexible and achieve competitive success as opportunities develop, competitors act, customer needs evolve and market dynamics change. This criterion also considers the vendor's history of responsiveness. Marketing Execution: The clarity, quality, creativity and efficacy of programs designed to deliver the organization's message to influence the market, promote the brand and business, increase awareness of the products, and establish a positive identification with the product/brand and organization in the minds of buyers. This "mind share" can be driven by a combination of publicity, promotional initiatives, thought leadership, word of mouth and sales activities. Customer Experience: Relationships, products and services/programs that enable clients to be successful with the products evaluated. Specifically, this includes the ways customers receive technical support or account support. This can also include ancillary tools, customer support programs (and the quality thereof), availability of user groups, service-level agreements and so on. Operations: The ability of the organization to meet its goals and commitments. Factors include the quality of the organizational structure, including skills, experiences, programs, systems and other vehicles that enable the organization to operate effectively and efficiently on an ongoing basis.
Completeness of Vision
Market Understanding: Ability of the vendor to understand buyers' wants and needs and to translate those into products and services. Vendors that show the highest degree of vision listen to and understand buyers' wants and needs, and can shape or enhance those with their added vision. Marketing Strategy: A clear, differentiated set of messages consistently communicated throughout the organization and externalized through the website, advertising, customer programs and positioning statements. Sales Strategy: The strategy for selling products that uses the appropriate network of direct and indirect sales, marketing, service, and communication affiliates that extend the scope and depth of market reach, skills, expertise, technologies, services and the customer base. Offering (Product) Strategy: The vendor's approach to product development and delivery that emphasizes differentiation, functionality, methodology and feature sets as they map to current and future requirements. Business Model: The soundness and logic of the vendor's underlying business proposition. Vertical/Industry Strategy: The vendor's strategy to direct resources, skills and offerings to meet the specific needs of individual market segments, including vertical markets. Innovation: Direct, related, complementary and synergistic layouts of resources, expertise or capital for investment, consolidation, defensive or pre-emptive purposes. Geographic Strategy: The vendor's strategy to direct resources, skills and offerings to meet the specific needs of geographies outside the "home" or native geography, either directly or through partners, channels and subsidiaries as appropriate for that geography and market.
© 2022 Gartner, Inc. and/or its affiliates. All rights reserved. Gartner is a registered trademark of Gartner, Inc. and its affiliates.
コメント